【佳學(xué)基因檢測】基因選擇:貝葉斯變量選擇方法
腫瘤基因檢測公司排名國內(nèi)熱點(diǎn)
與同行交流時醫(yī)學(xué)博士年度腫瘤匯報知悉《Bioinformatics》在.?2003 Jan;19(1):90-7.發(fā)表了一篇題目為《基因選擇:貝葉斯變量選擇方法》腫瘤靶向藥物治療基因檢測臨床研究文章。該研究由Kyeong Eun Lee?,?Naijun Sha,?Edward R Dougherty,?Marina Vannucci,?Bani K Mallick等完成。促進(jìn)了腫瘤的正確治療與個性化用藥的發(fā)展,進(jìn)一步強(qiáng)調(diào)了基因信息檢測與分析的重要性。
腫瘤轉(zhuǎn)移惡化的基因根源臨床研究內(nèi)容關(guān)鍵詞:
基因選擇,模式選擇,基因表達(dá),變量選擇
腫瘤靶向治療基因檢測臨床應(yīng)用結(jié)果
通過表達(dá)模式選擇重要基因是微陣列實驗中的一個重要問題。由于樣本量小和變量(基因)數(shù)量多,選擇過程可能不穩(wěn)定。本文提出了一種用于基因(變量)選擇的分層貝葉斯模型。腫瘤基因解碼課題組使用潛在變量將模型專門用于回歸設(shè)置,并在執(zhí)行變量選擇之前使用貝葉斯混合。腫瘤發(fā)生的基因原因追溯組通過在模型的維度(重要基因的數(shù)量)上分配先驗分布來控制模型的大小。參數(shù)的后驗分布不是明確的形式,腫瘤基因檢測需要結(jié)合使用截斷采樣和基于馬爾可夫鏈蒙特卡羅 (MCMC) 的計算技術(shù)來模擬來自后驗的參數(shù)。貝葉斯模型足夠靈活,可以識別重要基因并進(jìn)行未來預(yù)測。該方法通過 cDNA 微陣列應(yīng)用于癌癥分類,其中基因 BRCA1 和 BRCA2 與乳腺癌的遺傳傾向相關(guān),并且該方法用于鑒定一組重要基因。該方法也成功地應(yīng)用于白血病數(shù)據(jù)。
腫瘤發(fā)生與反復(fù)轉(zhuǎn)移國際數(shù)據(jù)庫描述:
Selection of significant genes via expression patterns is an important problem in microarray experiments. Owing to small sample size and the large number of variables (genes), the selection process can be unstable. This paper proposes a hierarchical Bayesian model for gene (variable) selection. We employ latent variables to specialize the model to a regression setting and uses a Bayesian mixture prior to perform the variable selection. We control the size of the model by assigning a prior distribution over the dimension (number of significant genes) of the model. The posterior distributions of the parameters are not in explicit form and we need to use a combination of truncated sampling and Markov Chain Monte Carlo (MCMC) based computation techniques to simulate the parameters from the posteriors. The Bayesian model is flexible enough to identify significant genes as well as to perform future predictions. The method is applied to cancer classification via cDNA microarrays where the genes BRCA1 and BRCA2 are associated with a hereditary disposition to breast cancer, and the method is used to identify a set of significant genes. The method is also applied successfully to the leukemia data.
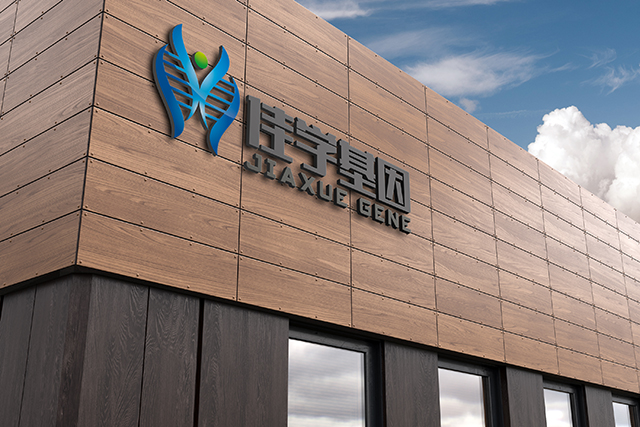
(責(zé)任編輯:佳學(xué)基因)